Biotechnology in an AI World
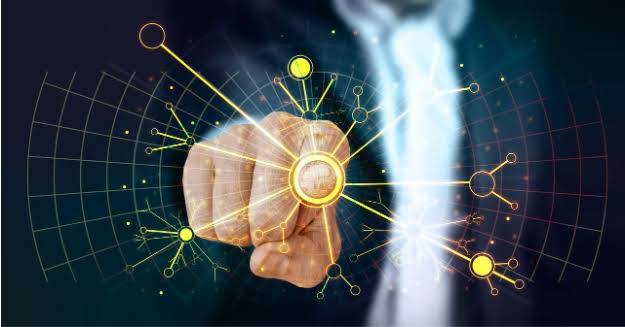
It’s a bit difficult to pin down exactly what people in the biotechnology industry mean by “artificial intelligence” (AI). In general, they seem content with a working definition, one that describes AI as a computer program that can learn and predict outcomes based on the data sets it receives.
Given that the working definition of AI is vague, it follows that the status of AI in the biotechnology industry is vague, too. For example, it is unclear whether AI is to be regarded as something new and revolutionary. Are news stories in the popular press any guide? These include breathless reports of how AlphaFold, an AI system developed by Google’s DeepMind, accurately predicted the structure of hundreds of thousands of proteins.
Although AlphaFold is a groundbreaking technology, it isn’t the be-all and end-all of AI in biotechnology. AI-related spadework in biotechnology is occuring in several fields of endeavor. Indeed, biotechnology companies far and wide have been implementing AI in their pipelines.
If we are to clarify AI’s status, we should begin by recognizing that AI in biotechnology hasn’t suddenly become mainstream. In fact, it is already mainstream. Moreover, it is diverse and ready to produce results. In the biotechnology industry, AI that is accurate, predictive, and productive is within reach. Such AI will be worth a hundred times its weight in bench lab scientists.
A vague definition of AI—big algorithms learning from big data sets—may be all we need. What’s really important is what AI systems do. And what AI systems do will depend on who is developing them.
Agilent Technologies, the instrumentation and consumables company, has taken steps into the AI world, including partnering with Visiopharm in AI-driven studies of cancer pathology. The companies see the work as a combination of different approaches.
Agilent’s perspective is well described by two of the company’s executives—Anya Tsalenko, PhD, computational biology section manager, and Stephen Laderman, PhD, director of research laboratories. They confirm that the most advanced AI approaches use computationally intensive models trained with large data sets.
“These models broadly fall into the category of machine learning and include different flavors of deep convolutional neural networks,” they say. “The successes of these new approaches are based on the combination of the power of modern computers; the ability to collect, store, and access large amounts of data; and the recent advances in computer science, mathematics, and statistics.”
“I totally understand that people define [AI] differently,” adds Carl Hansen, CEO of AbCellera Biologics, a Vancouver-based company using AI to create therapeutic antibodies. “And I think it’s really dangerous to talk about AI as a category. It’s way too abstract. In my opinion, there is a lot of very powerful and interesting applications of AI in drug discovery. And there’s also a lot of fluff.”
Yet another perspective is offered by Fabrice Chouraqui, PharmD, CEO of Cellarity, a Cambridge-based startup focusing on cell-based therapeutics derived from imaging- and machine learning–based approaches. He sees AI as a complement to existing paradigms, but one that hasn’t always lived up to past promises. “I think, today, AI is mainly used to improve existing approaches,” he elaborates.” So, the vast majority of the companies in drug discovery will tell you that they can leverage AI. By making the current discovery paradigm more efficient, they’ll tell you that they can use AI to find new targets.
“I have experience working with people who had, in the past, actually made claims that they couldn’t support. And not that they didn’t have the algorithm, to be fair. I think [the idea that the algorithm is all important] is perhaps one of the most common misunderstandings about AI. AI can only be as good as the quality of the data.”
Data sets aren’t the only source of biologically significant patterns. Such patterns can also be found in pictures of cells, tumors, and tissues—even if these pictures are analyzed using time-consuming, eye-strain-inducing, brute-force approaches. To find patterns in images more easily, researchers may take advantage of AI. According to Tsalenko and Laderman, AI image analysis can enhance both basic research and medical pathology.
“[We have] strong positions in live-cell microscopy and histopathology,” they explain. “With appropriate selection of methods and model training, AI image analysis enables one to locate, classify, count, and identify patterns in microscopy images, including those that are challenging for a human eye to see efficiently, comprehensively, and quantitatively.
“These attributes are among the drivers for the ongoing digital transformation of microscopy-based tissue analysis in clinical pathology laboratories. It is envisioned that in the end, AI will, for example, improve the precision with which cancer can be diagnosed and treated. In the case of live-cell imaging, AI can improve tracking of the dynamics and evolution of cells under study.”